Research
Make American Productivity Great Again – Background report
The slowdown of US productivity growth can be turned around if policymakers would launch an alternative policy agenda, focusing on stimulating investment in science, innovation and education.

Summary
Is the US losing its role as global technological leader?
In the post-WWII era, the US economy has been positioning itself as global technological leader. In 1990, the US had a leading role in the field of education by an arm’s length, but in 2017 it has been overtaken by many other OECD countries (Figure 1). The same is true for total R&D spending as a percentage of GDP (so-called R&D intensity): the US used to be one of the leading countries in R&D investment, but recently has made far less progress in raising growth than many other countries (Figure 2). A waning relative position in R&D spending does not seem to be limited to the US, but seems to be a pattern that applies to Anglo-Saxon countries in general. So obviously, one cannot solely blame the current US administration for sliding innovative capacity of the US economy, but we can’t say either that the Trump administration is making any efforts to reverse that trend. Moreover, it probably is aggravating the situation, given the (proposed) budget cuts in the fields of science, innovation and education.
Figure 1: The US used to be the frontrunner in the field of education

Figure 2: Little dynamics in US R&D spending

The consequence of sluggish investment in the field of innovation, education and science most likely has a direct link to US labor productivity woes (Figure 3). Average annual US productivity growth has slowed down from 1.8% over the period 1970-2010 to a meagre 0.4% between 2010 and 2018. To compare, in the Euro Area the productivity slowdown between 1996-2010 and 2010-2018 was only 0.2ppts annually. The large drop in the US is due to a combination of poor capital contribution of 0.1ppts and a substantial slowdown of TFP growth. TFP growth deteriorated from an average annual growth of 1.1% over the period 1969-2010 to 0.4% in 2010 to 2018.
Figure 3: Productivity and TFP growth have slowed down markedly since 2010
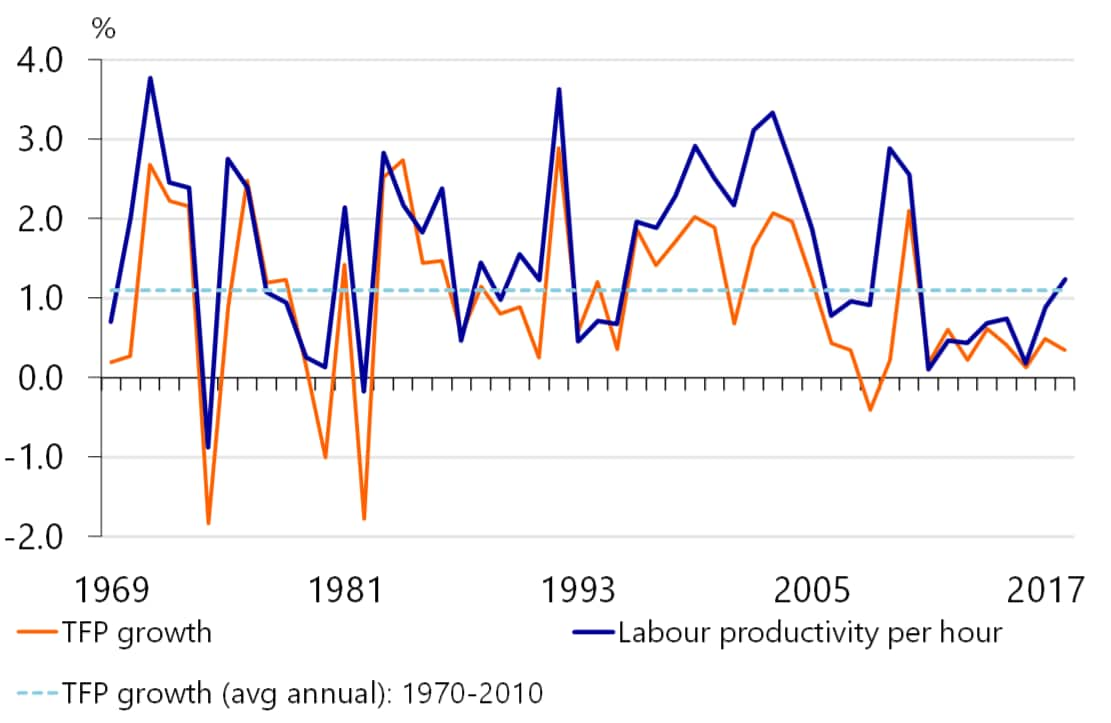
Supply-side economics: Generic tax cuts or targeting endogenous growth factors?
In the last two decades, US policymakers have not been giving science, innovation and education much attention. However, these factors are important pillars of US potential growth. Paul Romer, founding father of the endogenous growth theory, for which he received the Nobel Prize for Economics last year, has shown that growth of potential output is not an exogenous process, but requires investment in Research and Development (R&D) and human capital. Hence, US potential growth is not a completely autonomous process, but can be influenced by policy choices.
While previous administrations already weren’t making policy choices in favor of these growth drivers, the Trump administration is going one step further: it is slashing budgets for science, innovation and education. In the 2018 budget proposal, the administration aimed to reduce Research and Development (R&D) funding by 5%, but this figure is even positively biased, as it includes higher spending on defense R&D as well. In reality, the budget cuts for several public research bodies are much larger, with especially the Environmental Protection Agency (EPA) taking a hit. The 2019 Budget proposal was no different: NASA, the EPA, the National Institutes of Health, the National Science Foundation and various other institutions all face budget cuts in Trump’s plans. This year’s budget request from the Ministry of Education proposes to eliminate 29 programs, which embodies cuts of USD 8.5bn and lowers spending levels by 12% compared to fiscal 2019.[1]
The Trump administration has taken a different approach to stimulating the economy. Instead of targeting endogenous growth factors, a generic tax cut was the policy of choice. This is an approach that was advocated by ‘supply-side economists’ in the early 1980s, based on the assumption that slashing tax rates would on balance generate more tax revenue because the increase in the tax base (caused by higher economic activity as higher-than-optimal marginal tax rates are reduced) would outweigh the loss in tax revenue due to lower taxation. However, there is no empirical evidence that this assumption - the US economy operating on the downward-sloping segment of the Laffer curve - holds in reality. In fact, Trump’s massive tax overhaul which was implemented early 2017, the Tax Cuts and Jobs Act (TCJA), is proving to be a costly one: estimates of the amount of debt added over a time span of ten years center around USD 1500bn, as we will discuss below. So the generic tax cut will cost a lot of money, but is this the most efficient way to stimulate US economic growth? More specifically, the question we ask ourselves in this Special is: is the Trump administration making the best policy choices to boost the potential growth rate of the US economy, which is more important in the long run than a short-term boost to economic growth? In other words, which policy option would benefit the US economy the most?
We take a three-step approach in this study. First, to assess the supply side of the US economy we develop an endogenous framework which enables us to calculate the level of US potential growth. Second, we run a scenario analysis of what the impact on the US economy would have been if the Trump administration had launched an alternative plan focusing on innovation, science and education, rather than implementing the Tax Cuts and Jobs Act. Third, we examine if such a supply-side driven policy agenda would be effective in averting or mitigating the cyclical slowdown that we expect in 2020.
[1] Next to these proposed cuts in the education budget, the Trump administration proposed to scale back on the so-called Supporting Effective Instruction State Grants by USD 2.1bn, which focusses on supporting teacher salaries.
Step 1: Assessing US growth potential
Our first step is to set up a framework which we can use to forecast US potential GDP growth. Potential GDP growth is the growth of output that the US economy can sustain over the longer term without triggering substantial inflationary pressure. It is possible for an economy to grow above potential output for a while, but ultimately a situation of overemployment, overtime for workers and a higher than usual rate of utilization of machinery will generally lead to upward pressure on wages and capital compensation and will pull back the economy toward more sustainable growth levels.
Supply-side economics
The level of potential output of an economy is determined by the supply side of the economy, which is determined by labor inputs and labor productivity per hour (figure 4). Labor inputs can be broken down into the amount of persons employed and the amount of hours worked by each worker. Labor productivity per hour is determined by capital per hour, which encompasses IT capital (software, computers, mobile phones, etc.) and non-IT-capital (buildings, infrastructure, machines), and a residual factor: total factor productivity (TFP). TFP arguably is the purest indicator of technology, as it indicates how productive both capital and labor are in generating value added. There is a vast literature that has looked into the key factors of TFP (see Annex 1). Some important proven factors are Research & Development (R&D), human capital, domestic competition, technological catching-up, foreign competition through trade and entrepreneurship.
Figure 4: Decomposition of the supply side of the economy
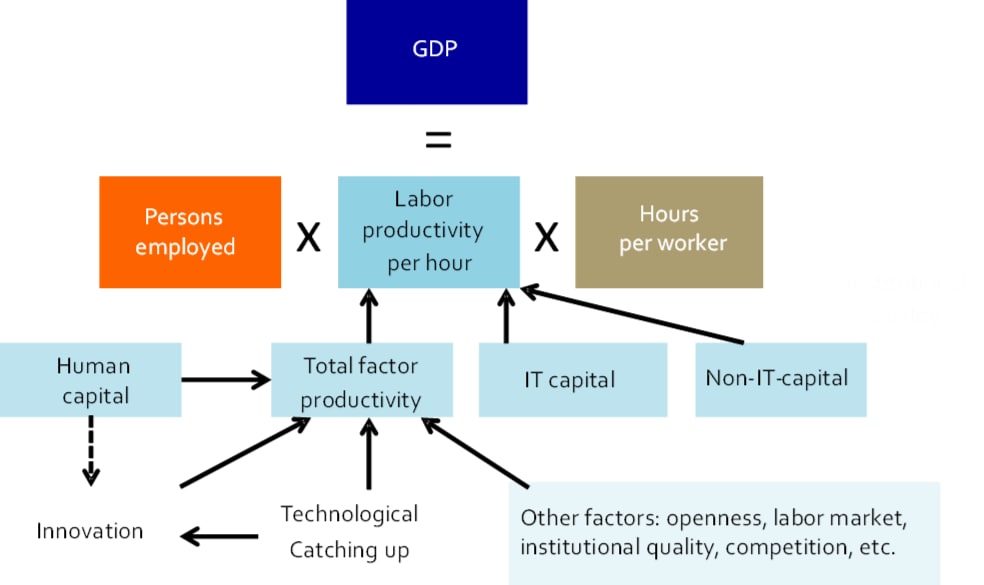
Extrapolating the supply-side components of growth
In order to forecast US growth potential, we need to extrapolate the underlying determinants. For capital and labor inputs, we use the econometric global trade model NiGEM to forecast the series up to 2030 (see Table A.3 in Annex A.4).
Extrapolating TFP, however, is complicated, given the large set of determinants and the variety in impact on TFP. Of course, one can simply extrapolate productivity trends, but we choose to forecast TFP growth by using an endogenous TFP framework. In Annex A.2 we present this framework, which is empirically estimated for the US in Annex A.3. Combined with the assumptions on all underlying drivers in Annex A.4, we are able to produce a fully endogenized path of US growth potential (Figure 5).
Findings
Our calculations show that, under current policies, the US has the potential to grow by 2.0% on an annual basis between 2019 and 2030. These findings are in line with those of the CBO (see red line in Figure 5), but the composition of growth is somewhat different (Table 1). The CBO expects the labor contribution to be 0.5ppts per annum up to 2029, whereas in our projections this component only contributes 0.3ppts. Our labor productivity forecast of 1.7%, on the other hand, is more optimistic than that of the CBO (1.4%).
Figure 5: US growth potential is roughly 2%

Table 1: Potential growth US (annual average, up to 2029)

Step 2: The impact of different policy options
Now that we have our fully endogenized supply-side framework in place, we can run scenarios what the impact of different policy options would be on the US economy. We run two scenarios. In our first scenario, we calculate the impact of a R&D stimulus package replacing the Tax Cuts and Jobs Act. In a second scenario, we assess the impact of a policy agenda focusing on actively stimulating human capital to levels of global frontrunner Germany. Before we can run these scenarios, however, we need to strip the effect from the TCJA from our baseline.
Stripping out the effect of the Tax Cuts and Jobs Act from the baseline
The Tax Cuts and Jobs Act was implemented in January 2017. According to initial calculations by the Tax Foundation, the Joint Committee on Taxation, and the Tax Policy Center, the Act will lower federal tax revenues by roughly USD 1500bn over ten years (2018-2027). The Congressional Budget Office (CBO) shows that the impact on the fiscal deficit could even be as high as USD 1800bn in ten years, including positive feedback effects. In 2018, revenues already fell short by 275bn vis-à-vis the projections of the CBO (see here).
Most analysts tend to agree that the TCJA is costly, but the question is what additional economic growth it generates. According to a thorough study by the Tax Foundation, the TCJA would increase GDP by an average of 0.3ppts annually over the next decade. On average, US GDP would arrive at 2.13% under the new tax regime compare to 1.84% under the previous circumstances. 2018 would see the largest impact of the tax reforms, raising GDP growth by 0.44ppts compared to the baseline. According to our own calculations, the corporate tax cut from 35% to 21% would boost GDP by 1.9ppts vis-à-vis the baseline, registering the largest impact in 2018 of 1.6ppts. We expect a limited impact on private consumption, as the largest cuts as a share of income is going to taxpayers in the 95th to 99th percentiles of the income distribution (see here). It is common knowledge that the wealthiest people have the lowest marginal propensity to consume.
If we shift our focus to the supply side of the economy, we have calculated that the impact of the TCJA results in a boost of capital contribution of 0.7ppts in 2018 vis-à-vis the baseline and an additional 0.3ppts in 2019. The impact in subsequent years is marginal at best. Moreover, we do not estimate a statistically and economically significant impact of lower corporate taxes on TFP growth (see Annex A.3). If we strip out the impact of the TCJA from our baseline, we get the results illustrated in Figure 6.
Figure 6: TCJA raises US growth by 1.0% in total

Scenario 1: Investing 1500bn in R&D
In this first scenario, we conduct a thought experiment in which we calculate the counterfactual of what would happen if the US administration had not chosen to cut taxes and instead had invested this money (i.e. USD 1500bn) into stimulating R&D.
The government could stimulate R&D expenditure directly by raising subsidies to research institutes and universities, or by giving financial incentives to the private sector to spend more on R&D. A common instrument is the use of R&D tax credits, which seems to be an effective way to stimulate business R&D expenditure. A wide range of international research shows that additional public resources allocated to R&D tax credits leads to higher private R&D spending. There is, however, no clear consensus on the size of this elasticity. Although some studies find a larger effects, most well-conducted studies indicate a value of less than 1. Judging from some meta-studies (summarized by the European Commission, 2014), an elasticity of 0.5 seems to be a valid point estimate. This is supported by more recent studies (e.g. Thomson, 2017). In this study, we therefore assume that an increase of USD 1000bn in tax credits would generate USD 500bn in additional private R&D expenditure.
Ultimately, we allocate USD 500bn of the costs of the TCJA to public R&D investment and allocate 1000bn by raising the corporate R&D tax credit, which results in additional private R&D spending of 500bn. Both impulses are distributed over 13 years (2018-2030), which raises private and public R&D capital as illustrated in Figure 7.
The gains in productivity from this alternative R&D policy agenda is illustrated in Figure 8. In scenario 1, US potential growth is raised by 6¼ppts between 2018-2030 against the baseline (which includes the effects of TCJA).
Figure 7: Private and public R&D capital in scenario 1

Figure 8: An alternative R&D policy agenda would raise potential growth cumulatively by 6%

Scenario 2: Closing the gap in human capital with Germany
In our second scenario we assume that the US, next to the R&D policy stimulus, will raise its policy efforts to close the gap to the global frontrunner in the field of education: Germany. Germany currently holds the top position in the education index by the UN (Figure 1), which is a combined index of the mean years of schooling and the expected years of schooling. But also on other education indicators, such as the PISA score, Germany outranks the US on all aspects (Figure 9).
Figure 9: Germany also outranks the US on the PISA scores

The human capital indicator that we have used in our TFP model is taken from the Penn World Tables, which combines the average years of education from Barro and Lee (2013) with information on the return of education based on Mincer equation estimates (Psacharopoulos, 1994). These data, however, are only available up to 2014, so we use more recent data from the UN education index to calculate the relative gap between the US and Germany, which is approximately 4% (see Figure 1). Next, in our scenario we assume that the US is able to close this gap in 8 years (between 2018 and 2026) and apply this path to the human capital index from the Penn World Tables. The result of this policy agenda is illustrated in Figure 10.
In scenario 2, higher productivity growth on the back of the impulse in R&D and human capital would result in additional economic growth of 14ppts in total, compared to our baseline scenario (Figure 11). So roughly speaking, an alternative education agenda would roughly double the GDP gains from scenario 1.
Figure 10: Human capital index in scenario 2

Figure 11: Alternative policy agenda would raise GDP growth by 14ppts cumulatively

Step 3: Assessing the mitigating impact of higher potential growth on a US recession
One of the upsides of higher potential growth is that it has a mitigating impact on negative cyclical swings as well. The line of reasoning here is simple: an economic bust due to weakness in aggregate demand is relative to the potential growth. A higher level of potential growth will therefore keep actual growth higher, and may even prevent it to go below zero.
In our most recent forecasts, we have penciled in a recession in the US in 2020 (Figure 12). This forecast is based on extensive research by RaboResearch on the movements of the yield curve and other cyclical indicators, such as declining investment in the residential sector.
To assess the mitigating impact of higher potential growth on the US recession, we use our macro-econometric trade model NiGEM to run a forecast of US GDP growth with the TFP trajectories of both scenario 1 and scenario 2 factored in.
Figure 12: The US recession in 2020

Figure 13: Mitigating impact on the US recession
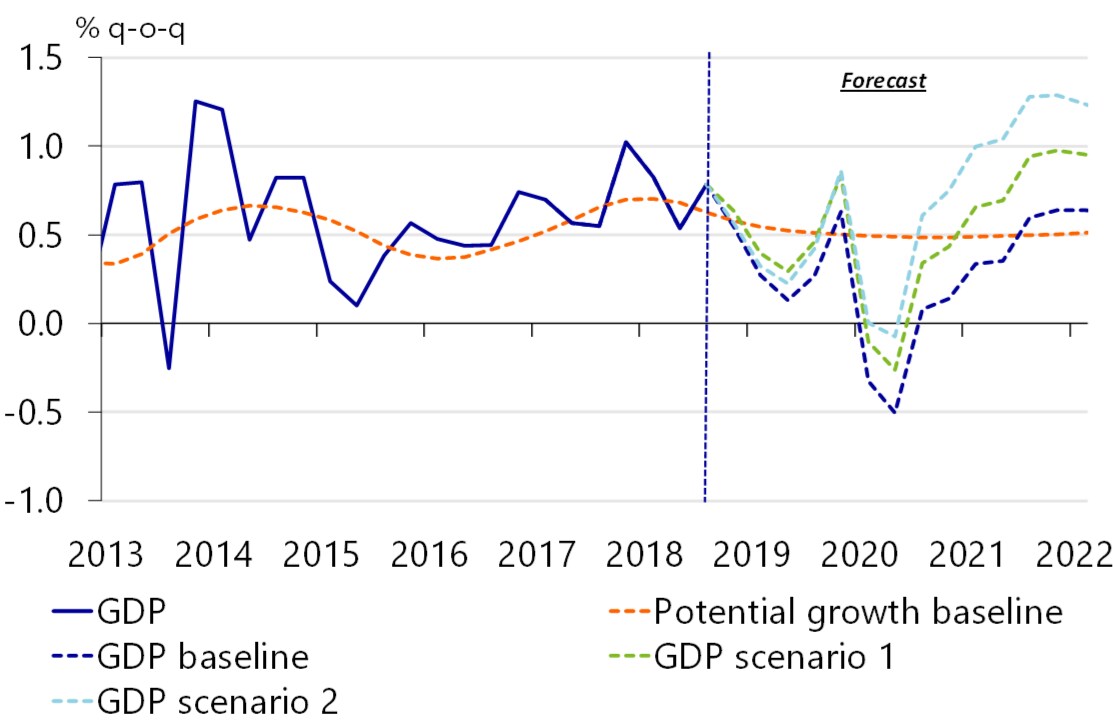
The results show that it will be very hard to avoid a recession in the US, even if the government were to launch the substantial supply-side stimulus incorporated in scenario 1 and 2 (Figure 13). However, the downturn would be substantially less deep: -0.8% cumulative in our baseline, -0.3% in scenario 1 and -0.1% in scenario 2. What is more important, the recovery from the bust would be much stronger in both scenarios compared to the baseline. Over the longer term, the differences in actual growth are equal to the already mentioned effects that apply to the supply side of the economy: 6¼ppts additional growth in scenario 1 compared to the baseline and 14ppts in scenario 2.
Final remarks
In this Special we argue that over the past administrations, US policymakers have shifted their focus too much to lifting short-term economic activity and boosting the stock market rather than stimulating the supply side of the US economy. This has resulted in a substantial slowdown of US productivity growth. The Trump administration is exacerbating this development by slashing budgets for science, innovation and education. Although generic tax cuts are providing relief in the short term, ongoing sluggish productivity growth is slowly eroding the core of the US economy. This will make the economy much more vulnerable to cyclical downturns in the long run. After all, if economic growth will continue to hover at low levels, only a modest headwind could push the economy into a recession.
We also claim that sluggish productivity development in the US is not an exogenous development, but something which is affected by the decisions of policymakers. All in all, economic policymaking is about incentives. A generic corporate tax cut, which was lowered from 35% to 21% under the TCJA, only partly translates in higher investment and has primarily boosted shareholder value. In contrast, an innovation and education agenda forces firms and public research bodies to allocate the funds to productivity-enhancing investments, but this policy route requires more patience, as the impact will take some years to materialize.
Our calculations show that fostering productivity growth by means of implementing a broad innovation, science and education agenda would lift US potential growth substantially. Investing the costs of the Tax Cuts & Jobs Act (of USD 1500bn) in a program fostering public and private R&D would raise potential growth by more than 6ppts cumulatively compared to the baseline (up to 2030). An additional policy agenda focusing on intensifying investment in education on top of R&D stimulus could even raise potential GDP growth by 14ppts.
More upsides than higher potential growth
The upsides of such a policy shift would not be limited to solely higher potential growth. First, raising potential GDP growth also mitigates the negative impact of a potential US downturn. Second, fostering economic growth by means of innovation would raise competitiveness of the American corporate sector, which provides second-order effects in terms of job opportunities for US workers in the long run. Third, an innovation and science policy agenda could be targeted to boost research on tackling (global) societal challenges in the field of, e.g., climate change, nutrition and health, food and water security and sustainable agriculture, clean and efficient energy, smart green and integrated transport, cyber security and counterterrorism. Fourth, policies focusing on potential GDP growth have higher returns to the government in terms of tax receipts, so in the end government debt will be lower. This is (in terms of % GDP) amplified further by the higher level of GDP (denominator effect). Lastly, the positive impact would stretch much further than US shores. It is well-known that countries can benefit from the others that are close to the technological frontier (Fagerberg, 1987; Camaron et al., 1998). This process is referred to as ‘technological catching-up’, and its impact is quite substantial. Erken (2008) calculated that between 1993 and 2002, the impact of catching-up towards the technological leader (i.e. the US) on TFP growth in the Australia, Denmark, Germany, and the Netherlands was almost 50% (Figure 14). In Belgium, France, Italy, New Zealand, Portugal, the UK, the impact was even larger than 50%. Indeed, over the past decades, US technologies and innovations reshaped every-day life across the globe, ranging from the laser, the internet, smartphones, personal computers to chemotherapy. Higher prosperity in other parts of the world due to US innovations leads to more export opportunities for US firms in the second round as well.
Knowledge spillovers from US innovations in the past have been the result of decisions by policymakers in the US determined to provide the best education and take the lead in public research in combination with US firms focusing on outpacing their competitors by means of investment in R&D to deliver the best products and services. At the moment, it could even be argued that sluggish investment by the US in science, innovation and education is one of the main culprits behind the slowdown of global productivity growth. And from this context, it becomes even more important for the US presidential election campaign, which will kick off next year, to focus on the key aspects that will shape the future of the US and global economy.
Figure 14: Catching-up has contributed heavily to productivity growth

Literature
Alcalá, F., and A. Ciccone (2004). Trade and productivity. Quarterly journal of economics, 119(2), 613-646.
Acs, Z.J., Estrin, S., Mickiewicz, T. and L. Szerb (2018). Entrepreneurship, institutional economics, and economic growth: an ecosystem perspective. Small Business Economics, 1-14.
Audretsch, D.B. (2018). Entrepreneurship, economic growth, and geography. Oxford Review of Economic Policy, 34(4), 637-651.
Barro, R.J. and J.W. Lee (2013). A new data set of educational attainment in the world, 1950–2010. Journal of Development Economics, 104, 184-198.
Bassanini, A. and S. Scarpetta (2002). Does human capital matter for growth in OECD countries? A pooled mean-group approach. Economics Letters, 74(3), 399–407.
Bassanini, A., Scarpetta, S. and P. Hemmings (2001). Economic growth: the role of policies and institutions. Panel data evidence from OECD countries. OECD Economics Department Working Papers, no. 283.
Belorgey, N., Lecat, R. and T.P. Maury (2006). Determinants of productivity per employee: An empirical estimation using panel data. Economics Letters, 91(2), 153–157.
Bloom, N., Draca, M. and J. Van Reenen (2016). Trade induced technical change? The impact of Chinese imports on innovation, IT and productivity. Review of Economic Studies, 83(1), 87-117.
Bourlès, R. and G. Cette (2007). Trends in “structural” productivity levels in the major industrialized countries. Economics Letters, 95(1), 151–156.
Braunerhjelm, P., Acs, Z.J., Audretsch, D.B. and B. Carlsson (2010). The missing link: knowledge diffusion and entrepreneurship in endogenous growth. Small Business Economics, 34(2), 105-125.
Buccirossi, P., Ciari, L., Duso, T., Spagnolo, G. and C. Vitale (2013). Competition policy and productivity growth: An empirical assessment. Review of Economics and Statistics, 95(4), 1324-1336.
Cameron, G., Proudman, J. and S. Redding (2005). Technological convergence, R&D, trade and productivity growth. European Economic Review, 49(3), 775-807.
Cameron, G., Proudman, J. and S. Redding, S. (1998). Productivity convergence and international openness. In J. Proudman & S. Redding (Eds.), Openness and growth (pp. 221–260). London: Bank of England.
Carree, M.A., Van Stel, A.J., Thurik, A.R. and A.R.M. Wennekers (2002). Economic development and business ownership: An analysis using data of 23 OECD countries in the period 1976–1996. Small Business Economics, 19(3), 271–290.
Carree, M.A., A.J. Van Stel, A.R. Thurik and A.R.M. Wennekers (2007). The relationship between economic development and business ownership revisited. Entrepreneurship and Regional Development, 19(3), pp. 281-291.
Carree, M.A. and A.R. Thurik (2008). The lag structure of the impact of business ownership on economic performance in OECD countries. Small Business Economics, 30(1), 101–110.
Coe, D.T., & Helpman, E. (1995). International R&D spillovers. European Economic Review, 39(5), 859–887.
Coe, D.T., Helpman, E. and A.W. Hoffmaister, A. W. (2009). International R&D spillovers and institutions. European Economic Review, 53(7), 723–741.
Cohen, W.M. and D.A. Levinthal (1989). Innovation and learning: the two faces of R&D. The Economic Journal, 99(397), 569–596.
Cohen, D. and M. Soto (2007). Growth and human capital: good data, good results. Journal of Economic Growth, 12(1), 51-76.
Donselaar. P. (2011). Innovatie en productiviteit. Het Solow-residu ontrafeld (in Dutch). Erasmus University Rotterdam.
Edwards, S. (1998). Openness, productivity and growth: what do we really know? The Economic Journal, 108(447), 383-398.
Engelbrecht, H.J. (1997). International R&D spillovers, human capital and productivity in OECD economies: An empirical investigation. European Economic Review, 41(8), 1479–1488.
Erken (2008). R&D, Productivity and Entrepreneurship. ERIM, Erasmus University Rotterdam.
Erken, H.P.G., P. Donselaar and A.R. Thurik (2016). Total factor productivity and the role of entrepreneurship. Journal of Technology Transfer, 43(6), 1493-1521.
European Commission (2014). A study on R&D tax incentives. European Commission Taxation papers No. 52.
Fagerberg, J. (1987). A technology gap approach to why growth rates differ. Research Policy, 16(2–4), 87–99.
Frantzen, D. (2000). R&D, human capital and international technology spillovers: A cross-country analysis. Scandinavian Journal of Economics, 102(1), 57–75.
Griffith, R., Redding, S. and J. Van Reenen (2004). Mapping the two faces of R&D. Productivity growth in a panel of OECD industries. Review of Economics and Statistics, 86(4), 883–895.
Griliches, Z. (2000). R&D, education, and productivity. A retrospective. Cambridge (MA): Harvard University Press.
Guellec, D. and B.P. Van Pottelsberghe de la Potterie (2004). From R&D to productivity growth: Do the institutional settings and the source of funds of R&D matter? Oxford Bulletin of Economics and Statistics, 66(3), 353–378.
Himmelberg, C.P. and B.C. Petersen (1994). R & D and internal finance: A panel study of small firms in high-tech industries. Review of Economics and Statistics, 38-51.
Khan, M. and K.B. Luintel (2006). Sources of knowledge and productivity: How robust is the relationship? OECD, STI/Working Paper 2006/6.
Lee, G. (2005). International R&D spillovers revisited. Open Economies Review, 16(3), 249-262.
Lucas, R.E. (1988). On the mechanisms of economic development. Journal of Monetary Economics, 22(1), 3–42.
Michelacci, C. (2003). Low returns in R&D due to the lack of entrepreneurial skills. The Economic Journal, 113(484), 207–225.
Park, W.G. (1995). International R&D spillovers and OECD economic growth. Economic Inquiry, 33(4), 571-591.
Prieger, J.E., Bampoky, C., Blanco, LR. and A. Liu (2016). Economic growth and the optimal level of entrepreneurship. World Development, 82, 95–109.
Psacharopoulos, G. (1994). Returns to investment in education: A global update. World development, 22(9), 1325-1343.
Romer, P.M. (1990). Endogenous technological change. Journal of Political Economy, 98(5), S71–S102.
Thomson, R. (2017). The effectiveness of R&D tax credits. Review of Economics and Statistics, 99(3), 544-549.
Van Elk, R., B. Verspagen, B. Ter Weel, K. Van der Wiel and B. Wouterse (2015). A macroeconomic analysis of the returns to public R&D investments. CPB Netherlands Bureau for Economic Policy Analysis, 313
Annex A.1: drivers of TFP
There is a vast academic literature on determinants of TFP (see for an overview Erken, Donselaar and Thurik, 2016). Below we give a brief description of these determinants.
Human capital and R&D
Human capital[2] and R&D are the most important driving forces behind technological progress and TFP, not only from a theoretical perspective (see Lucas, 1988; Romer, 1990), but also from an empirical point of perspective: see Griffith et al. (2004), Engelbrecht (1997), Bassanini and Scarpetta (2002), Cameron et al. (2005) and Erken, Donselaar and Thurik (2016).
Domestic R&D expenditure is an important driver of TFP growth, not only to generate domestic innovations, but also to benefit from knowledge developed abroad. A country needs so-called ‘absorptive capacity’ to be able to understand foreign technologies and internalize these in domestic innovation processes (Cohen and Levinthal, 1989). R&D can be conducted by firms (private R&D) as well as by public knowledge institutes and universities (public R&D). There is much evidence that private R&D generates positive productivity effects (see e.g. Coe et al., 2009; Coe and Helpman, 1995). With respect to public R&D, the evidence is mixed. Guellec and Van Pottelsberghe de la Potterie (2004) find a positive effect of public R&D capital, whereas Khan and Luintel (2006) and Van Elk et al. (2015) have difficulties reproducing these results. In this study, we also examine the impact of US public R&D capital on productivity growth.
Knowledge developed abroad is an important source for domestic innovation and productivity development. Foreign R&D activities on the other hand affect innovation of domestic firms only when absorptive ability is taken into account, which is in line with the findings of Cohen and Levinthal (1989). In this study we mainly examine the role of the import quote as a conduit for foreign knowledge spillovers, which is in line with the approach by Coe and Helpman (1995), Lee (2005) and Cameron et al. (2005).
It is pretty evident that at the current junction, many countries benefit much more from knowledge development in the US as global technological leader than the other way around. The US nevertheless can benefit from trade with other countries for domestic innovation and productivity purposes as well. Openness to foreign trade fosters market competition, which stimulates firms to reduce their X-inefficiencies and increase efforts to innovate. Bloom, Draca and Van Reenen (2016) for instance find evidence that Chinese import competition has increased technical change within European firms (within effect) and also caused a shift of employment towards technologically more advanced firms (sector composition effect). Other studies that find a robust direct positive effect of international trade on productivity are by Edwards (1998) and Alcalá and Ciccone (2004).
Other factors
Entrepreneurship is an important determinant of productivity. Inspired by the limitations of the endogenous growth theory, Braunerhjelm et al. (2010) have developed different models that introduce a filter between knowledge in general and economically-relevant knowledge; they identify entrepreneurship as a mechanism that reduces this so-called ‘knowledge filter’.[3] Bottom-line is that entrepreneurs are needed to valorize knowledge. There is also empirical evidence of a positive relationship between entrepreneurship and productivity (e.g. Carree and Thurik, 2008; Erken, Donselaar and Thurik, 2016) or economic growth (e.g. Acs et al., 2018; Audretsch, 2018), although some find that the relationship between entrepreneurship and economic growth rather is U-shaped (e.g. Carree et al. 2002; Prieger et al. 2016) or L-shaped (Carree et al., 2007).
Labor input generates adverse TFP effects (Belorgey et al., 2006; Bourlès and Cette, 2007; Erken, Donselaar and Thurik, 2016). High labor participation is often characterized by increased deployment of less-productive labor, which lowers labor productivity. Working fewer hours may have a positive impact on productivity if less fatigue occurs among workers or if employees work harder during the shorter number of active hours. Another factor that affects total factor productivity are business cycle effects, as labor and capital endowments are not immediately adjusted to business cycle volatility, which makes TFP susceptible to fluctuations of the business cycle. Firm profitability supposedly has a positive impact on TFP. More profits support higher R&D expenditure by firms (Himmelberg and Petersen, 1994). In addition, higher profit expectations can motivate firms to innovate at a higher rate (given a fixed amount of R&D capital). Lastly, higher profits provide firms with financial means to stimulate innovation (given a fixed degree of R&D capital). We use the capital income share as a proxy to capture the profitability effect. The capital income share is defined as gross capital income as a percentage of the gross value added of businesses. The negative counterpart of profitability is taxation. Taxation could have a negative impact on productivity: a higher rate of taxation implies negative incentives in certain markets and less incentive to innovate, which consequently could result in a less efficient economy.
[2] Human capital is defined as quality improvement of labor due to education and training.
[3] The idea behind the knowledge filter is that entrepreneurship serves as a conduit for knowledge. R&D by itself is not a growth safeguard, just as fostering entrepreneurship is insufficient for propelling growth. Entrepreneurs have to exploit knowledge (R&D) in order to lead to positive growth. This conclusion is also drawn by Michelacci (2003), who develops an endogenous growth model where innovation requires the matching of an entrepreneur with a successful invention.
Annex A.2: A framework for empirical TFP analysis
In order to model all mechanisms addressed above, we have adopted the framework used by Erken, Donselaar and Thurik (2016). Under the neoclassical conditions of perfect competition in product markets and constant returns to scale in the production factors of capital and labor, the marginal products of capital and labor are equal to the return on capital and the wage rate, respectively. It can be derived that, in that case, the output elasticities of capital and labor are equal to the shares of capital income and labor income in total factor income. The annual growth of TFP can then be calculated as follows:

where Y denotes gross domestic product, K and L denote (physical) capital input and labor (measured in physical units such as hours worked) and ωK is the share of capital income in total factor income, or stated differently, the share of capital income in the gross domestic product. Moreover, Δ denotes mutation in first differences, i stands for country, t is a time index (i.e. year) and log represents the natural log. From (1), we can define our baseline TFP model as:

where α1 measures the effect of growth of domestic R&D capital (S) on TFP growth. The coefficient α2 picks up the effect of foreign R&D capital (Sf). Foreign R&D is interacted with the import quote, which is defined as the import volume (M) as a ratio of gross domestic product volume (Y). R&D capital is calculated by using the perpetual inventory method on intramural R&D expenditure and taking into account a depreciation of capital of 15% due to the obsolescence of knowledge. This depreciation rate is often used to calculate R&D capital, based on Griliches (2000, p. 54), who refers to this percentage as the ‘‘conventional’ 15 percent figure for the depreciation of R&D-capital’. Nominal R&D expenditure is deflated using an index for the price of R&D, which consists for 50% of a deflator for domestic expenditure and 50% of an wage index. Foreign R&D is measured by a proxy of R&D in 19 other OECD countries, weighted by bilateral import shares for domestic R&D capital.
The term α3 measures the impact of human capital (H). The average years of (tertiary) education are usually used as an indicator to measure the amount of human capital in a country (see Barro and Lee, 2013). Cohen and Soto (2007) claim to produce better data than Barro and Lee, as they use information from surveys based on uniform classification systems of education over time, and an intensified use of information by age groups. However, the Penn World Tables 9.0 from which we take the data on human capital, adopts several criteria in order to assess which human capital series to use for which county (see here) and we directly follow their approach. Moreover, when adopting the Barro and Lee data series, the Penn World Table also takes into account the return of education based on Mincer equation estimates (see Psacharopoulos, 1994).
Lastly, coefficient α4 is a vector containing different control variables, such as openness of the economy, public R&D capital, entrepreneurship, labor inputs and the business cycle. Two notions are important in this respect. First, to measure openness of the economy, we first use trade exposure[4] as an indicator (see Bassanini et al., 2001), but as a second step adjust trade export data for country size using estimations by Donselaar (2011). Small economies are by definition more exposed to foreign trade, regardless of their trade policy or competitiveness. Second, high-quality entrepreneurship data from the COMPENDIA dataset is only available up to 2009. This forces us to extend the series based on the OECD self-employment series, which goes at the expense of the quality of our entrepreneurship variable. For all technicalities on variable construction, we refer to Erken (2008), Donselaar (2011) and Erken, Donselaar and Thurik (2016).
[4] Trade exposure is defined as: TRADE = X + (1-X) x M, where X represents the ratio of exports in relation to GDP, M is the ratio of imports in relation to domestic demand. Domestic demand is calculated by domestic production minus exports plus imports.
Annex A.3: A TFP model for the United States
Our approach to model the impact of R&D, human capital and foreign knowledge development on productivity within a dynamic setting fits a broad strand of literature (see Park, 1995; Frantzen, 2000; Griffith et al., 2004; Cameron et al., 2005; Buccirossi et al., 2013). Table A.1 shows the variables that we use in our US TFP model, as well as their data sources. We use simple OLS to estimate the model and use the Akaike, Schwarz criterion and Hannan-Quinn information criterion to obtain the optimal lag structure. The estimation results are shown in Table A.2.
Our baseline estimation is illustrated in column (1) of Table 3 and consists of domestic R&D capital, foreign R&D capital interacted with the import share and human capital. All variables show a statistically significant impact on TFP growth. Domestic private R&D capital (coefficient c1) shows a coefficient 0.18, which means that a 1% higher growth of R&D conducted by firms on US soil generates a TFP growth of 0.18ppts. As coefficient (c2) is an interaction variable, the interpretation is somewhat complicated. Our results show that if foreign R&D capital increases by 1%, US domestic TFP growth will increase by 0.06ppts, whereas if the import quote rises by 1%, this adds another 0.12ppts to US TFP growth. Finally, the human capital index has a positive effect on TFP growth: the coefficient (c3) shows that an increase of the human capital index by 1% yields higher US TFP growth by 1.5ppts.
Table A.1: Description of variables and data sources US TFP model

Table A.2: Estimation results US TFP growth model

In column (2) we add public domestic R&D capital (c4), which fails to show a significant impact. This is in line with many studies that have difficulties assessing a significant impact of public R&D (alongside private R&D capital) on economic growth (see for an overview Van Elk et al. (2015)). However, when we add openness of the economy and a business cycle variable in column (3), public R&D shows a statistically significant impact on TFP growth. The coefficient is 0.49, which means that a 1% increase in public domestic R&D capital leads to additional TFP growth of 0.49ppts. Moreover, the two controls (openness (c5) and the business cycle (c6)) also have a statistically significant and positive impact on TFP growth and the explanatory power of the model jumps markedly (R2 rises from 0.21 to 0.51).
In column (4), we add two labor input variables: participation (c7) and hours worked per worker (c8). As expected, the participation variable has a (statistically significant) negative impact on TFP growth. The reason is that a higher growth of labor participation generates a composition effect which serve as a drag on productivity growth, as often the additional people that start working are less productive and skilled than the already participating ones. The amount of hours worked per worker does not have a significant impact on labor productivity growth. This also makes sense, as the US is one of the few OECD countries which is able to combine higher levels of productivity with high participation ratios and many hours worked per worker. In many countries there is a clear trade-off between hours worked and productivity per hour, but the US is an outlier in this respect.
In column (5) we add entrepreneurship (c9) as a control variable, which has a significant impact on TFP growth. This means that if the deviation of the business ownership rate from the equilibrium levels growth, TFP growth increases as well, as there are more people able to valorize knowledge. Reversely, if a country lacks this mechanism, there is a TFP growth penalty.
Finally, in columns (6) and (7), we subsequently add two variables related to the effect of firm profitability on productivity, which are and corporate tax as a % of GDP (c10) and the capital income quote (c11). Corporate tax fails to show a statistically significant effect. We have experimented with a different lag structure, construction of the corporate tax variable and the combination with other variables, but none of these experiments yield fruitful results. Although we know that via the route of additional corporate investment, lower corporate taxes (such as the Tax Cuts and Jobs Act) will have a positive impact on productivity growth, we seriously doubt if there is an additional effect of lower corporate taxes on total factor productivity growth. The capital income quote has a significant impact and a coefficient of 0.10, which means that an improvement of the capital income quote by 1% results in an increase in TFP growth by 0.10ppts.
Ultimately, our preferred equation is equation (7), which has a solid fit (see Figure A.1). We use this model to construct our benchmark scenario for the US and calculate TFP effects in our two alternative policy options scenarios.
Figure A.1: Rabobank US TFP model has a solid fit

Annex A.4: Baseline assumptions
US-China trade war
The developments in the US-China trade war has implications for our US baseline trajectory. In our current baseline, we assume that the US and China will be able to reach a trade deal somewhere in the upcoming months (most likely June). In this deal, we can expect China to show commitment to import more LNG, F&A and vehicles/planes from the US and even aiming to closing the trade deficit with the US, which is approximately USD 350bn on an annual basis. On paper, China will probably agree to give in to some of the US’ demands on more structural issues, such as forced transfer of technology, Intellectual Property (IP) violation, progress on opening up certain parts of the economy and cease excessive support of state-owned enterprises. In return, we expect that the US is willing to lower a small proportion of the tariffs that are currently in place. However, we expect that the protectionist packages from 2018 will be largely retained, as the US wants to keep pressure on China to keep its end of the bargain. This is even more likely, as China will not be keen to agree to an enforcement mechanism to monitor progress on implementing structural reforms. Ultimately, we expect that China will not be willing and able to follow up on US demands on structural issues and expect that the trade deal will break down eventually. Inefficient Chinese state-owned enterprises simply cannot weather more foreign competition. Consequently, tensions over trade will most likely flare up in the medium term.
Table A.3: Assumptions baseline
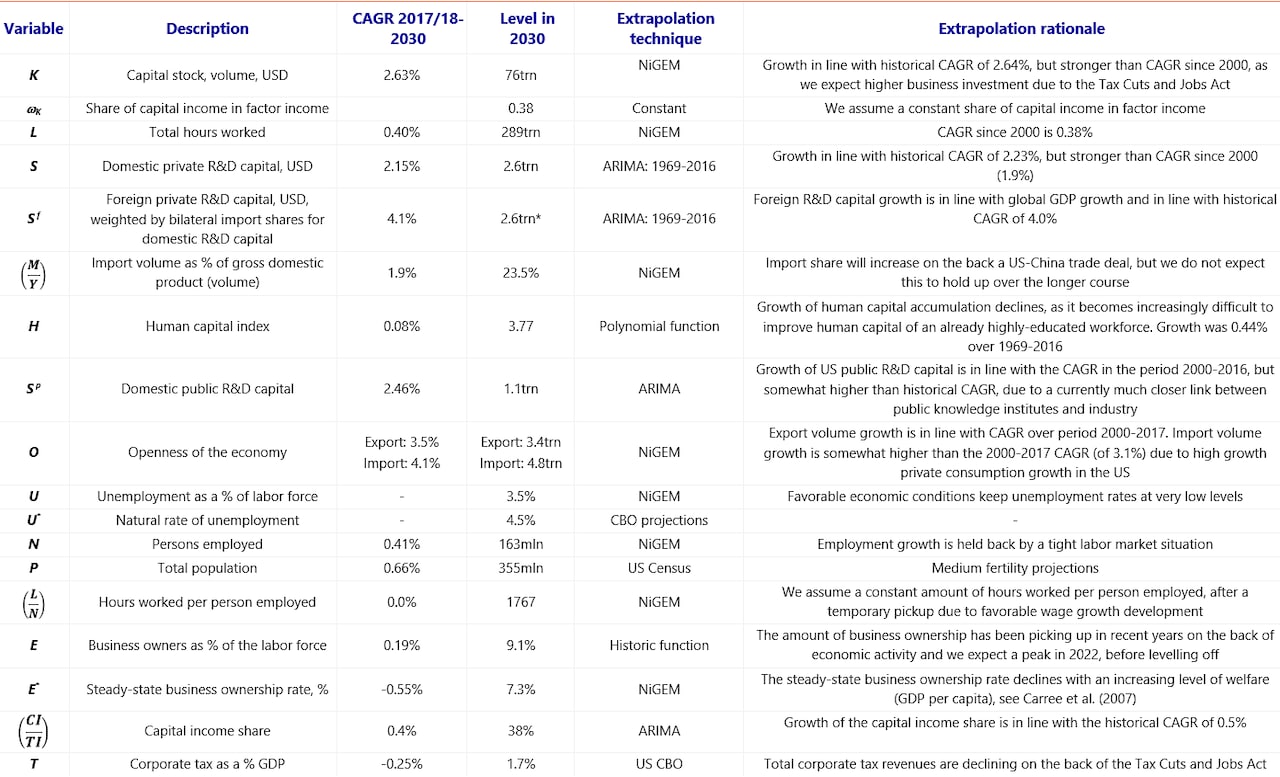